Author: Tami Forrester
I chose to look at one dataset that showed the race distribution by city council districts in 2010, based on information from the census. Unfortunately, it was presented in pdf form, which limited interactivity, though I found it interesting that of a total population of 6.5 million, white people accounted for around 81 percent of them, and led the population totals in all but three districts – Districts 4, 5 and 7. After looking at the dataset, I thought of the following questions
- Neighborhoods vs Districts? Could these be mapped out?
Looking through this table and other datasets left me confused as to how city council districts compared or related to neighborhoods. According to a link on the City of Boston website, the districts are mapped out as so:
I was also able to find another map showing crowdsourced neighborhood boundaries based on a survey.
I tried to overlay the two images to see if it would make for an easy comparison, though it it mostly confusing to look at.
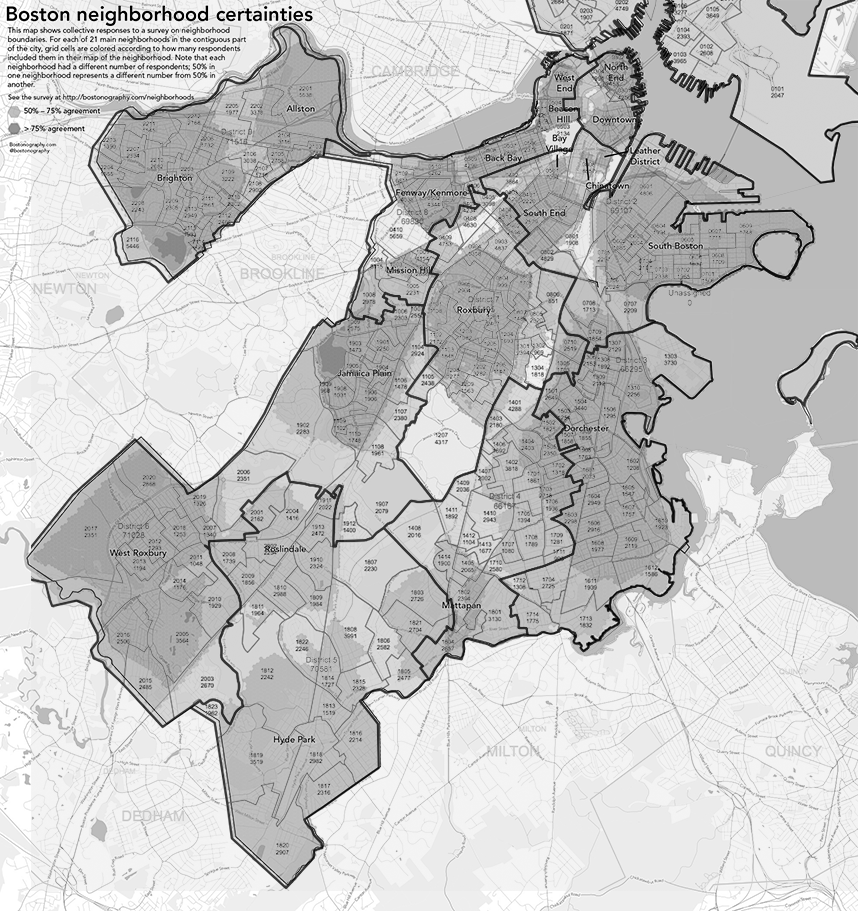
I also searched through datasets on the City of Boston site, but most only contained data about neighborhoods, and didn’t show relationships between them and districts. I was able to find a document on the City of Boston site, which compared the racial distribution over both districts and neighborhoods. However, trying to convert this data into an interactive form proved very tedious because it was locked in a pdf. Even after using an online tool to convert pdfs to excel spreadsheets, the formatting made it difficult to work with in Tableau.
- How have these demographics changed over time?
Another google search led me to yet another pdf of data showing how racial demographics have changed for specific years 1990, 1993 and 2002. I wasn’t sure why the specific years were chosen, and I didn’t try to analyze this in tableau, but was able to look over and see trends. I found it interesting that the amount of people identifying as various races in 1993 and 2002 was exactly the same both years, though the distribution over all districts in each time were different. For example, 140,305 people identified as black in both 1993 and 2002. The amount of black people per district was not the same between both years, however.
- What are some characteristics of the different districts?
Two characteristics I looked at specifically listed the public schools in Boston, and the crime incidents as reported by Boston police in 2012. Unfortunately, the schools were not mapped to their zones, but the crime incidents also included the zip-code and region area they were reported in. Using Tableau, I mapped the number of incidents that were reported in a particular region, and created a pie chart with neighborhoods mapped to the percentage or incidents reported.
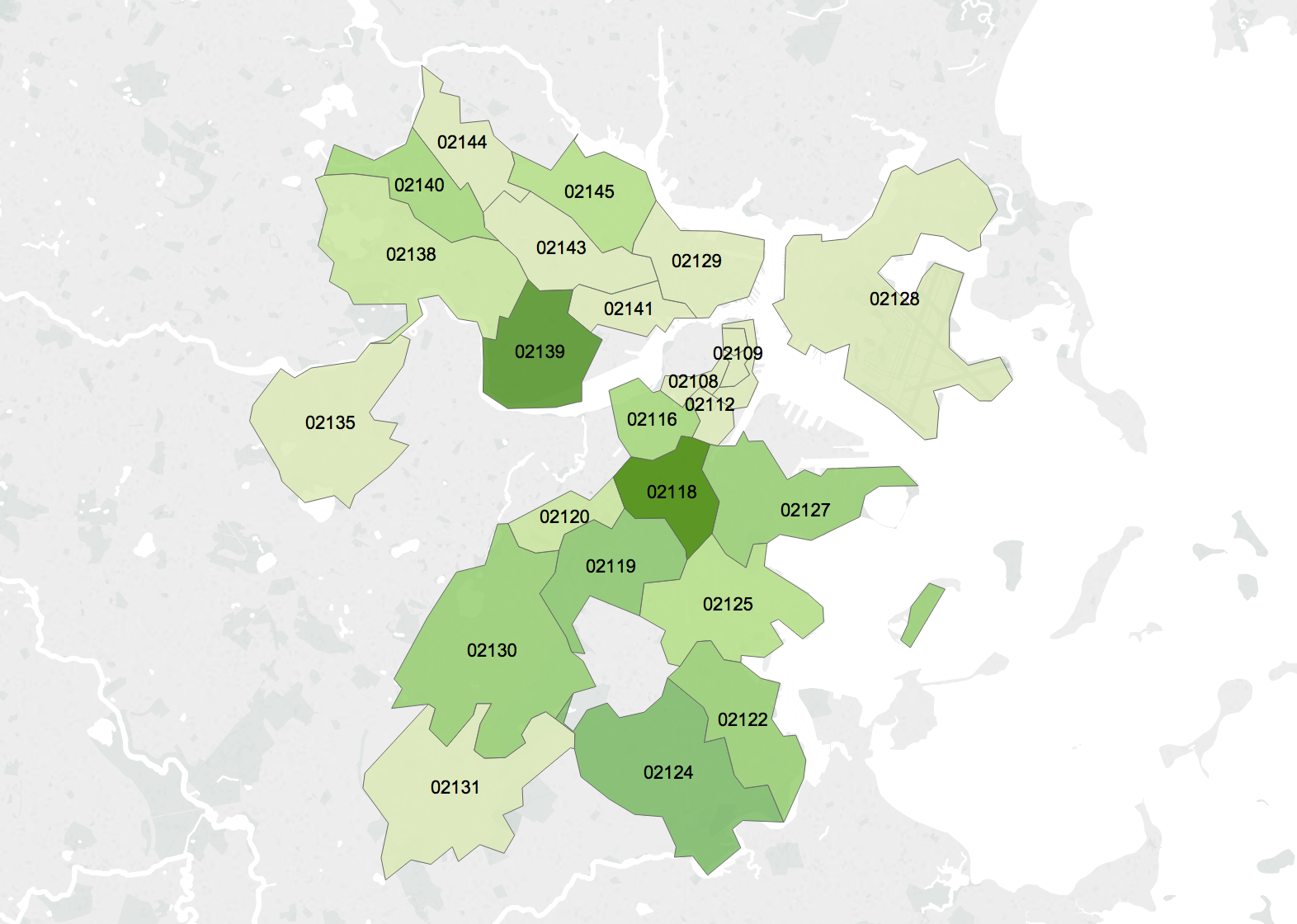
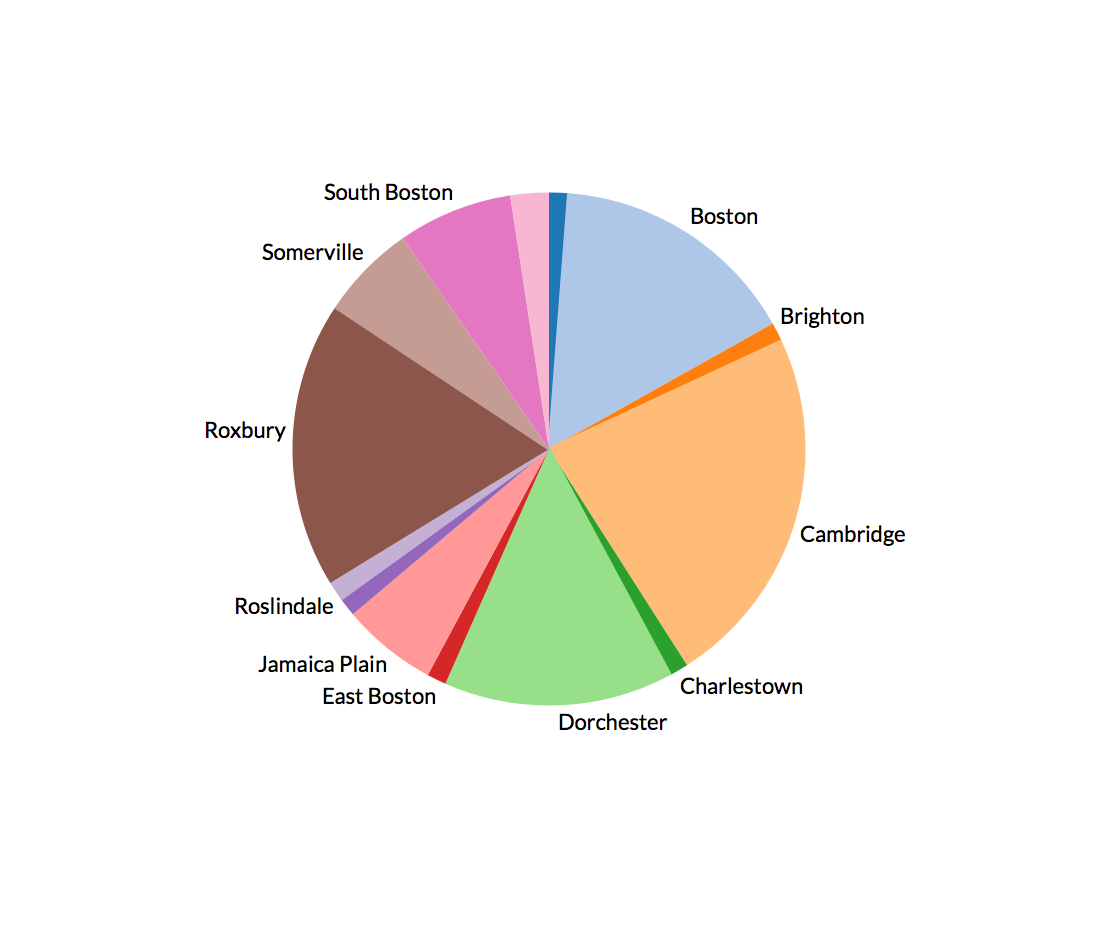
The crime incident reports also had a field called “reptdistrict”, which was presumably another metric used to characterize a particular region, though it was unclear what it meant.