Goal & Audience
Our goal is to inform the general public about the reality of food insecurity and build empathy and understanding. In particular, our game focuses on how SNAP benefits work and how it “feels” to use them in a real world setting – the grocery store. We acknowledge it is impossible to fully capture and simulate the entire experience of food insecurity, but our hope is that by placing the participant in the setting of a grocery store, which many people take for granted, we can introduce unfamiliar experiences to a familiar setting.
Game
Our game takes place in a small grocery store that we have set up, akin to Shaw’s. When the participants enter, we ask them the following questions:
1.How much food do you buy at a time? (1, 2, 3, or 4 weeks)
2.How many people are you shopping for?
We then ask them to do their shopping based on those answers. When they are ready to “check out,” we total up the prices of what they’ve bought. Then, based on how many weeks of food they are buying and how many people they are shopping for, we tell them how much the USDA Thrifty Food Plan (and by extension, SNAP) allocates for them to use, as well as the average SNAP allotment in Massachusetts. See our data here.
We then ask them, what would you give up to get down to the Thrifty Food Plan allotment? What would you give up if you SNAP was your only budget for money? Based on this, we ask them to put food back. This experience, of consciously deciding what to give up, and physically putting it back, can simulate the budgetary constraints food people on SNAP face daily, and help build empathy.
Finally, we take pictures of the before and after carts. A collection of these pictures will be shown to the participant at the end of the experience.
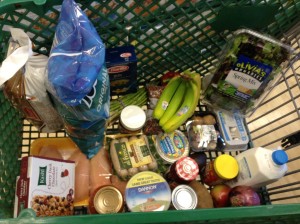
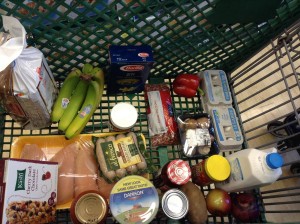
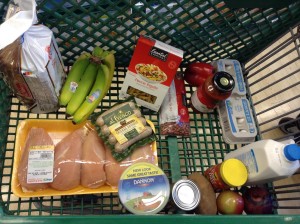
Notes
We tested out the idea on ourselves at a local Shaws, though given more time, we would have liked to try it out on a general audience and use prices that are not as high as the Cambridge Shaws. If we were to deploy a real version of this game, we would like to set up our own mini grocery store, but if that is not possible, then consider doing it at a real grocery store (though we haven’t thought through the details of that).
Here’s the data for what’s in our carts.
Weeks of groceries | |||||
People In Household | Maximum Monthly Allotment (Thrifty Food Plan) | 1 Week | 2 Weeks | 3 Weeks | 4 Weeks |
1 | $194 | $49 | $97 | $146 | $194 |
2 | $357 | $89 | $179 | $268 | $357 |
3 | $511 | $128 | $256 | $383 | $511 |
4 | $649 | $162 | $325 | $487 | $649 |
5 | $771 | $193 | $386 | $578 | $771 |
6 | $925 | $231 | $463 | $694 | $925 |
7 | $1,022 | $256 | $511 | $767 | $1,022 |
8 | $1,169 | $292 | $585 | $877 | $1,169 |
Weeks of groceries | |||||
People in Household | Massachusetts Average Monthly Allotment | 1 Week | 2 Weeks | 3 Weeks | 4 Weeks |
1 | $122.86 | $30.72 | $61.43 | $92.15 | $122.86 |
2 | $245.72 | $61.43 | $122.86 | $184.29 | $245.72 |
3 | $368.58 | $92.15 | $184.29 | $276.44 | $368.58 |
4 | $491.44 | $122.86 | $245.72 | $368.58 | $491.44 |
5 | $614.30 | $153.58 | $307.15 | $460.73 | $614.30 |
6 | $737.16 | $184.29 | $368.58 | $552.87 | $737.16 |
7 | $860.02 | $215.01 | $430.01 | $645.02 | $860.02 |
8 | $982.88 | $245.72 | $491.44 | $737.16 | $982.88 |